The predictive power of smartphone imaging to increase sustainability of crop production
Traditional crop monitoring methods are often labor-intensive and subjective or require expensive equipment. This project develops an AI-driven smartphone solution for detailed 3D/4D crop analysis, empowering farmers to enhance field management and sustainable practices.
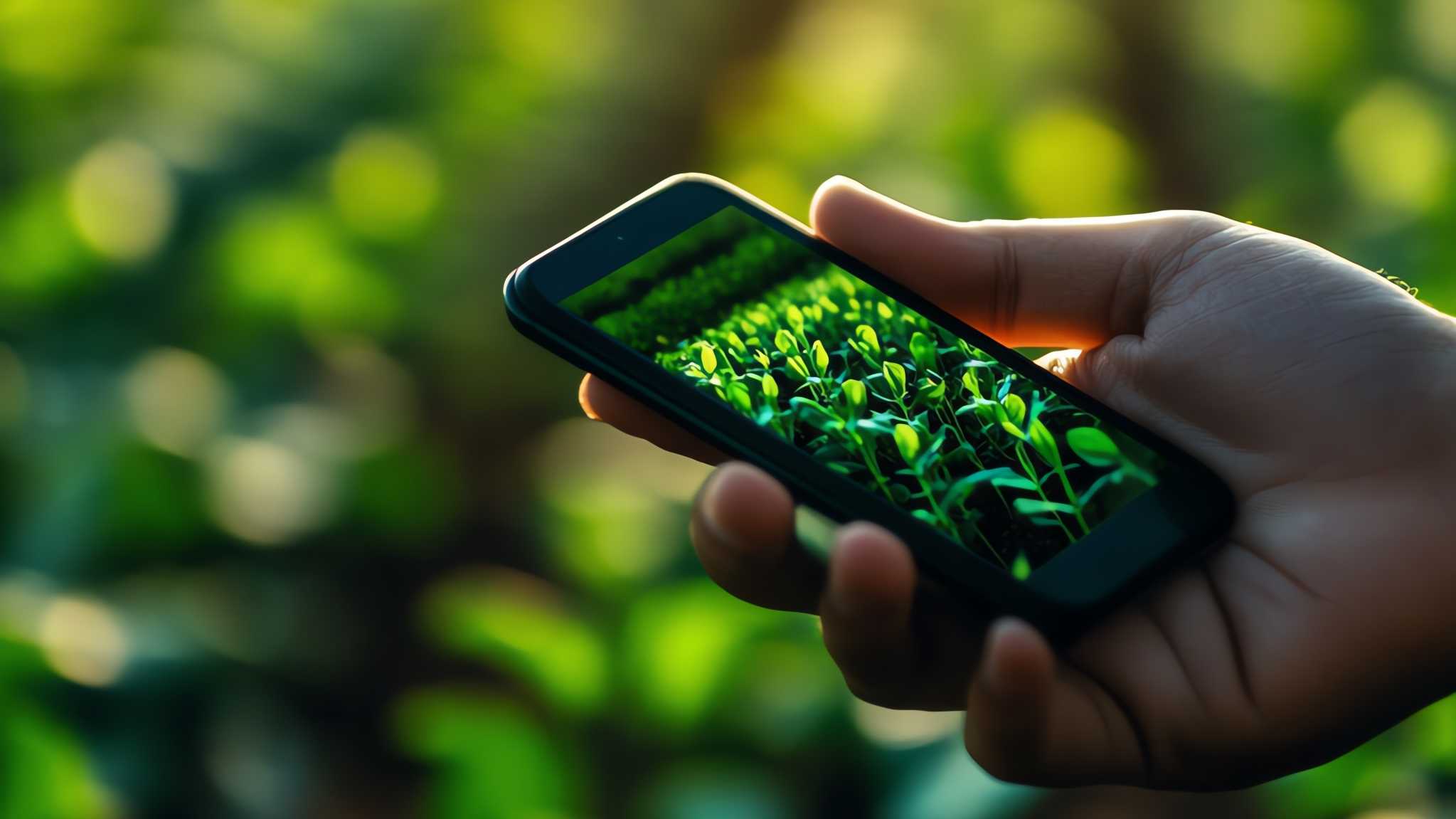
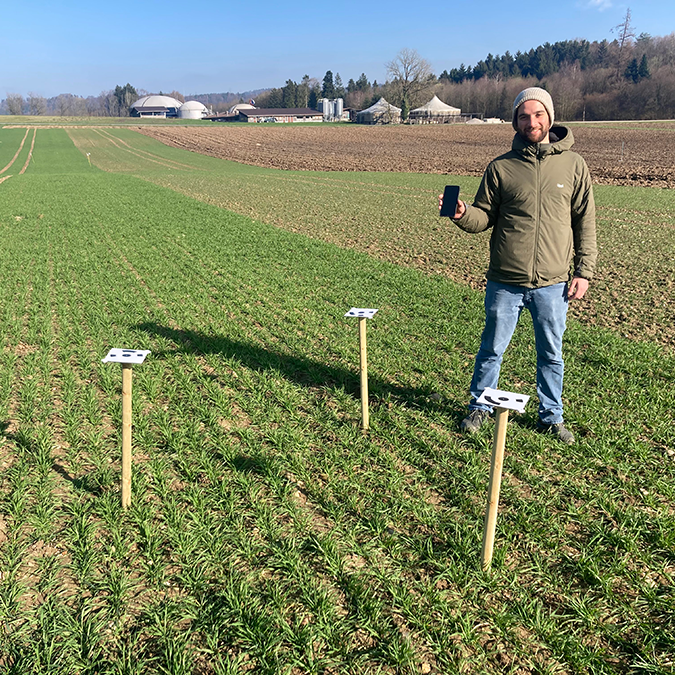
Project Duration: 2023-2026
Principal Investigator: Prof. Dr. Achim Walter, Crop Science Group, ETH Zurich
Co-Investigator: Dr. Michele Volpi, Swiss Data Science Center, ETH Zurich
Doctorate: Joaquin Gajardo Castillo, Crop Science Group, ETH Zurich
Postdoctoral Researcher: Dr. Lukas Roth, Crop Science Group, ETH Zurich
The primary goal of this project is to develop and validate new methods for extracting detailed crop growth and development information from multi-view images captured with a smartphone. To achieve this, the research focuses on advancing 3D reconstruction techniques to improve plant modeling in field conditions, developing 4D modeling approaches that allow tracking of crop growth over time, and creating robust algorithms for the automated measurement of key morphological traits. Additionally, the project explores how extracted traits can be integrated into crop growth models and ensures the accuracy and reliability of these methods through validation in real-world agricultural settings.
Accurate monitoring of crop growth and development is fundamental to making informed field management decisions. This interdisciplinary project explores innovative methods in computer vision, computer graphics, and artificial intelligence (AI) to enhance crop monitoring and phenotyping in agriculture. By leveraging advancements in 3D reconstruction and temporal modeling, the researchers aim to develop automated techniques that accurately measure crop growth and development traits from multi-view images. These techniques will enable the tracking of key plant characteristics such as height, leaf area, and wheat head size development in field environments. While the initial focus is on wheat, the overarching goal is to develop a generalizable technology applicable to various crops, thereby improving automated crop monitoring and supporting data-driven agricultural management.